Machine learning phases of matter
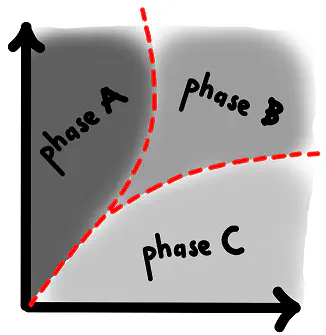
Short description
Identifying phase transitions and classifying phases of matter is central to understanding the properties and behavior of a broad range of material systems. While many-body systems have a large number of degrees of freedom, their phases are usually characterized by a small set of physical quantities like response functions or order parameters. However, the identification of phases and the transitions between them generally involves a large state space, requiring lots of intuition and knowledge about the underlying physical system. In this project, we develop and analyze machine-learning methods to perform these tasks in a data-driven manner. Such methods can aid in the identification of unexplored phase transitions as well as the discovery of new phases of matter.