Performance bounds for quantum control
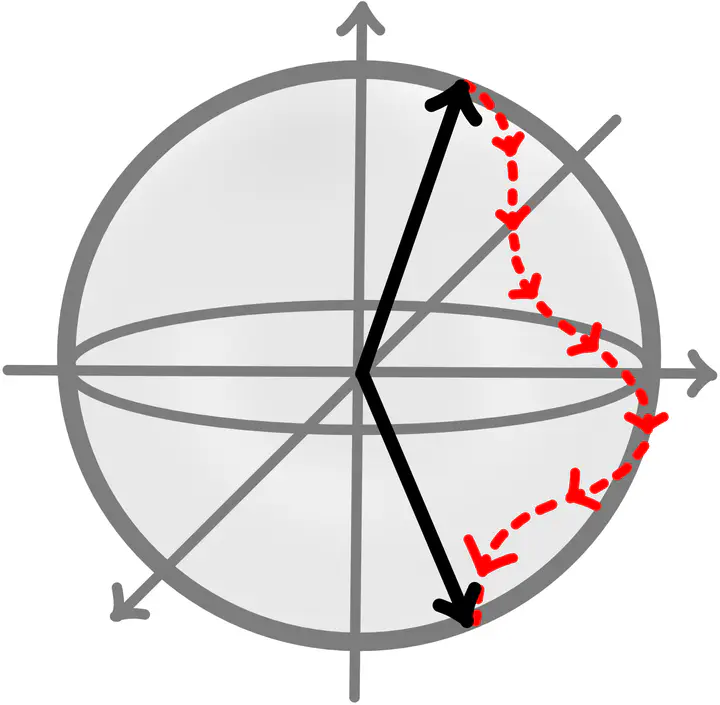
Short description
Feedback control of devices at the quantum level holds enormous potential for current and future applications in the field of quantum information science. However, due to the nonlinear and stochastic nature of quantum systems under continuous observation this is a difficult task. As such, heuristics, such as reinforcement learning or expert intuition, are often used for the design of quantum feedback controllers. While heuristically derived control policies are frequently found to perform remarkably well in practice, their degree of suboptimality essentially always lacks quantification. In this project, we tackle this problem by combining quantum filtering theory and moment-sum-of-squares techniques to construct a hierarchy of convex optimization problems that furnish monotonically improving, computable bounds on the best attainable performance for a large class of quantum feedback control problems.