Machine learning molecular collisions
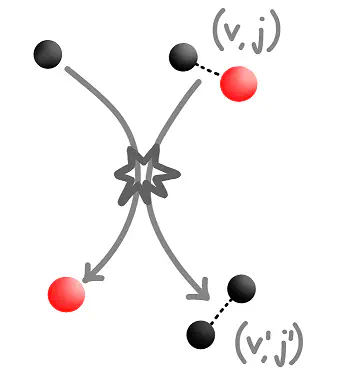
Short description
Predicting the outcomes of chemical reactions is one of the essential tasks for efficient material design and engineering. However, the exhaustive characterization of reactions at the microscopic state-to-state level quickly becomes computationally intractable using conventional approaches due to the rapid growth of the underlying state space. This is particularly restricting for the study of reactive gas flow at high temperatures, such as hypersonic flow around a space vehicle reentering the atmosphere, because a large number of states and transitions between them are accessible under such conditions. In addition, experimental data is scarce and the system is inherently nonequilibrium. In this project, we tackle this problem by training coarse-grained neural network-based surrogate models given data from ab initio reference simulations which predict the changes in energy and state population distributions upon collision.